The importance of data-driven decision making in business management – Data-driven decision making in business management isn’t just a buzzword; it’s the key to unlocking sustainable growth and outperforming competitors. In today’s hyper-competitive landscape, relying on gut feeling alone is a recipe for disaster. Instead, leveraging the power of data allows businesses to make informed choices, optimize operations, and ultimately, achieve a significant competitive edge. This article dives deep into why embracing data-driven strategies is no longer optional, but essential for survival and thriving in the modern business world.
From identifying profitable market segments to streamlining supply chains and personalizing customer experiences, data provides invaluable insights that can transform every aspect of a business. We’ll explore how to effectively harness data, overcome common challenges, and ultimately measure the tangible ROI of this transformative approach. Get ready to unlock the potential of your data and propel your business to new heights!
Defining Data-Driven Decision Making in Business
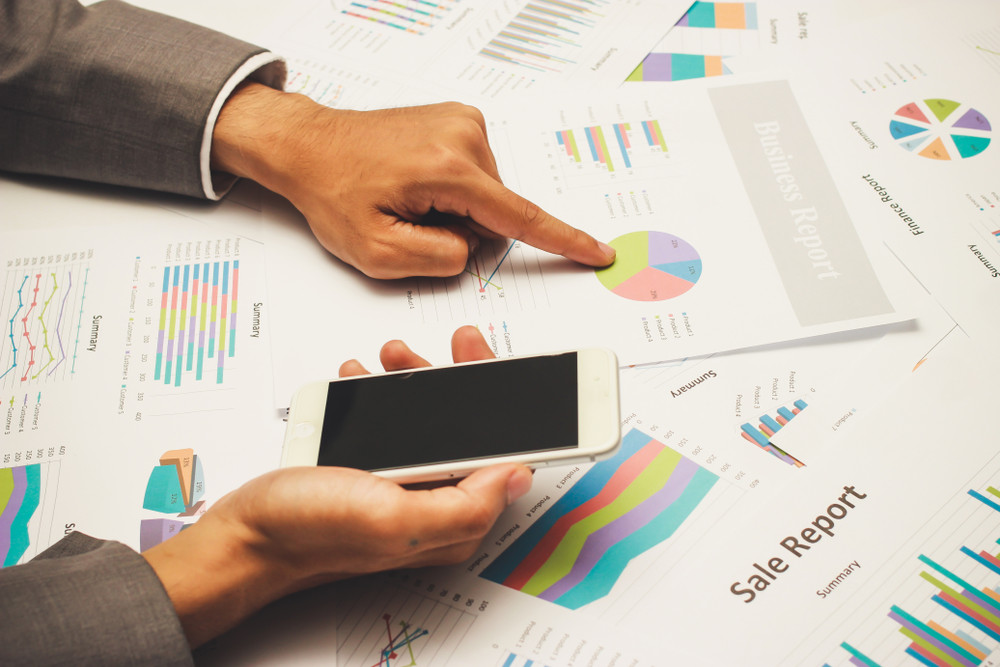
Data-driven decision making (DDDM) is the process of using data analysis and insights to inform business decisions, rather than relying solely on intuition or gut feeling. It’s about leveraging the power of information to make smarter, more strategic choices that ultimately lead to improved business outcomes. This approach moves beyond guesswork and allows for a more objective and measurable approach to problem-solving and opportunity identification.Data-driven decision-making hinges on several core principles.
Data-driven decision-making is crucial for navigating the complexities of modern business. Effective strategic planning relies heavily on accurate insights, and overcoming common pitfalls is key to success. For a deeper dive into refining your strategic approach, check out this helpful guide on overcoming common challenges in strategic business planning process. Ultimately, by addressing these challenges head-on, businesses can leverage data to make smarter, more profitable decisions.
Firstly, it prioritizes the collection and analysis of relevant data. Secondly, it emphasizes the use of robust analytical techniques to uncover meaningful patterns and trends within that data. Thirdly, it fosters a culture of experimentation and continuous improvement, using data to evaluate the effectiveness of decisions and refine strategies over time. Finally, it requires clear communication of data insights to stakeholders, ensuring everyone understands the rationale behind decisions.
Examples of Data-Driven Businesses
Many successful businesses have embraced data-driven approaches with impressive results. Netflix, for example, uses data analysis to personalize recommendations, improving user engagement and retention. Their sophisticated algorithms analyze viewing history, ratings, and other data points to suggest shows and movies users are likely to enjoy. This approach has been instrumental in their global success. Similarly, Amazon leverages vast amounts of customer data to optimize pricing, inventory management, and personalize the shopping experience.
Analyzing purchase history, browsing behavior, and search queries allows them to anticipate customer needs and tailor their offerings accordingly. These are just two examples of how data-driven strategies can fuel significant growth and market dominance.
Comparison of Traditional and Data-Driven Decision-Making
Traditional decision-making often relies heavily on experience, intuition, and anecdotal evidence. While valuable, this approach can be subjective and prone to bias. Data-driven decision-making, in contrast, emphasizes objective analysis of quantitative data. Consider a scenario where a marketing team needs to decide which advertising campaign to run. A traditional approach might involve a gut feeling based on past experiences or the team’s preferences.
A data-driven approach, however, would involve analyzing the performance of previous campaigns, examining customer demographics and behavior, and using statistical modeling to predict the likely success of different options. The data-driven approach offers a more scientific and less biased way to reach a decision.
Hypothetical Scenario Illustrating the Benefits of a Data-Driven Approach
Imagine a small coffee shop struggling with low sales during weekday afternoons. A traditional approach might involve guessing at the problem (e.g., poor marketing, bad coffee, inconvenient location). A data-driven approach, however, would involve collecting data on customer traffic, sales figures, and competitor activity. By analyzing this data, the shop owner might discover that the low sales are specifically linked to a lack of lunchtime offerings.
Armed with this insight, the shop could introduce a new lunchtime menu, perhaps offering quick and affordable sandwiches or salads. The resulting increase in sales and customer satisfaction would directly demonstrate the power of using data to make informed decisions, highlighting the superior efficiency and effectiveness of a data-driven approach over traditional guesswork.
Identifying Relevant Data Sources for Business Management
Data is the lifeblood of any successful business. Making informed decisions isn’t about gut feeling; it’s about leveraging the power of data to understand your market, your customers, and your internal operations. Identifying and utilizing the right data sources is the crucial first step in building a truly data-driven organization. This involves understanding both the internal and external sources available and ensuring the data you collect is both reliable and relevant.
Businesses today sit on a goldmine of information. However, raw data is meaningless without context and proper analysis. This section explores various internal and external data sources, emphasizes the importance of data quality, and Artikels methods for collecting and integrating data from diverse sources to create a comprehensive picture of your business landscape.
Internal Data Sources and Their Significance
Internal data sources offer a rich tapestry of information about your business’s performance and operations. This includes data generated from within your organization, providing valuable insights into your internal processes, customer interactions, and operational efficiency. Effective utilization of these sources can significantly enhance decision-making accuracy and efficiency.
Examples include sales data (transaction history, customer segmentation, sales trends), marketing data (website analytics, campaign performance, customer engagement), operational data (production metrics, inventory levels, supply chain data), financial data (revenue, expenses, profitability, cash flow), and human resources data (employee performance, turnover rates, skills inventory). Analyzing these data points together can reveal hidden patterns and opportunities for improvement.
External Data Sources and Their Value
Beyond internal data, external sources offer a broader perspective, providing insights into market trends, competitor activities, and the overall economic environment. This broader context is essential for strategic planning and anticipating future challenges or opportunities. These external data sources can be just as valuable, if not more so, than internal data in certain circumstances.
Examples of external data sources include market research reports, industry publications, economic indicators (inflation rates, unemployment rates, GDP growth), competitor analysis (market share, pricing strategies, product launches), social media analytics (brand sentiment, customer reviews, competitor mentions), and government data (census data, regulatory information). Understanding the external environment allows businesses to adapt and remain competitive.
Data Quality and Reliability: A Cornerstone of Effective Decision Making
The adage “garbage in, garbage out” rings especially true in data-driven decision-making. The quality and reliability of your data directly impact the accuracy and usefulness of your insights. Inaccurate or incomplete data can lead to flawed decisions with potentially severe consequences.
Ensuring data quality involves several steps, including data validation (checking for accuracy and completeness), data cleaning (removing inconsistencies and errors), and data standardization (applying consistent formats and definitions). Regular data audits and robust data governance procedures are essential for maintaining data integrity and building trust in your insights. Data reliability is often linked to the source; reputable sources generally provide more reliable data.
Methods for Data Collection and Integration
Collecting and integrating data from diverse sources requires a strategic approach. This involves choosing the right tools and techniques to gather, clean, and combine data from various systems and platforms.
Common methods include using enterprise resource planning (ERP) systems, customer relationship management (CRM) systems, data warehousing, and data integration platforms. These systems facilitate data collection, storage, and analysis, enabling businesses to gain a unified view of their data. Furthermore, data visualization tools are critical for transforming raw data into actionable insights.
Comparison of Data Sources
Data Source | Strengths | Weaknesses | Data Type |
---|---|---|---|
Internal Sales Data | Detailed transaction history, accurate sales figures | Limited external context, may not reflect market trends | Quantitative |
External Market Research Reports | Broader market perspective, industry trends | Can be expensive, may lack specific company details | Qualitative & Quantitative |
Social Media Analytics | Real-time customer feedback, brand sentiment | Data can be biased, difficult to quantify | Qualitative |
Government Economic Indicators | Reliable macroeconomic data, large-scale trends | May lag behind real-time changes, not company-specific | Quantitative |
Data Analysis Techniques for Business Insights
Unlocking the true potential of data requires more than just collecting it; it demands sophisticated analysis to unearth actionable insights. This involves employing various statistical and visualization techniques to transform raw data into strategic business decisions. The right analytical approach can significantly improve efficiency, boost profitability, and provide a competitive edge.
Descriptive Statistics for Business Overview
Descriptive statistics provide a foundational understanding of your data. These methods summarize the main features of a dataset, offering a snapshot of key performance indicators (KPIs). Common techniques include calculating measures of central tendency (mean, median, mode) and dispersion (range, variance, standard deviation). For instance, analyzing the average customer purchase value can reveal opportunities for upselling or targeted marketing campaigns.
Similarly, understanding the standard deviation in sales figures across different regions helps in identifying areas needing attention or those performing exceptionally well. These initial analyses form the basis for more complex investigations.
Statistical Inference and Hypothesis Testing
Statistical inference moves beyond simple description to draw conclusions about a larger population based on a sample. Hypothesis testing allows businesses to assess the validity of claims or assumptions. For example, a company might hypothesize that a new marketing campaign will increase website traffic. By comparing website traffic before and after the campaign launch using a t-test, they can determine if the observed increase is statistically significant or simply due to random variation.
This rigorous approach ensures that decisions are not based on speculation but on statistically sound evidence. A well-designed A/B test, for example, can rigorously compare the performance of two different marketing approaches, informing future strategies based on statistically significant results.
Regression Analysis for Predictive Modeling
Regression analysis is a powerful tool for building predictive models. It helps identify relationships between variables, allowing businesses to forecast future outcomes. For instance, a retail company might use regression analysis to predict future sales based on historical data, including factors like seasonality, advertising spend, and economic indicators. This allows for proactive inventory management and optimized resource allocation.
The resulting model can be used to predict sales for upcoming periods, allowing the company to adjust its production and marketing plans accordingly. Different regression models, like linear or multiple regression, can be applied depending on the complexity of the relationships between the variables.
Data Visualization for Effective Communication
Data visualization translates complex data into easily understandable charts and graphs. This is crucial for effective communication of insights to stakeholders. For example, a bar chart can clearly illustrate sales performance across different product categories, while a line chart can show trends in customer acquisition over time. A heatmap could highlight geographical areas with high customer concentration. Consider a scenario where a company uses a geographical heatmap to visualize customer density.
This instantly reveals areas with high concentration, informing strategic decisions on store placement or targeted marketing campaigns. The visual representation makes complex data instantly understandable, enabling quicker and more informed decision-making.
Step-by-Step Guide: Analyzing Customer Churn
Let’s analyze customer churn, a common business problem.
- Define the Problem: Clearly state the objective – to identify factors contributing to customer churn.
- Gather Data: Collect relevant data, including customer demographics, purchase history, customer service interactions, and churn status (yes/no).
- Clean and Prepare Data: Handle missing values, outliers, and inconsistencies. This might involve removing irrelevant data points or imputing missing values using appropriate statistical methods.
- Perform Analysis: Use descriptive statistics (e.g., churn rate, average tenure of churned customers) and potentially logistic regression to identify factors significantly correlated with churn.
- Visualize Results: Create charts (e.g., bar charts showing churn rates by customer segment, scatter plots illustrating relationships between variables) to communicate findings effectively.
- Interpret Results and Recommend Actions: Based on the analysis, identify actionable insights and recommend strategies to reduce churn (e.g., targeted loyalty programs, improved customer service).
Implementing Data-Driven Decisions Across Business Functions
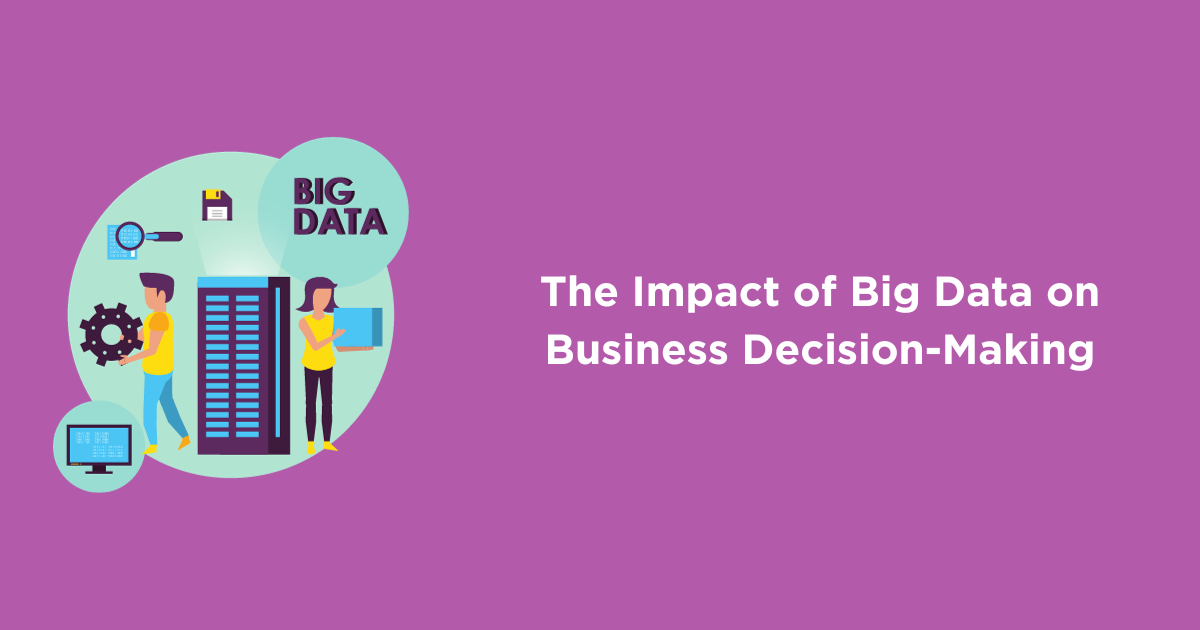
Data-driven decision-making isn’t just a buzzword; it’s the backbone of modern business success. By systematically collecting, analyzing, and interpreting data, companies can move beyond gut feelings and make informed choices across all departments, leading to increased efficiency, profitability, and a stronger competitive edge. This section explores how data-driven strategies transform key business functions.
Data-driven decision-making is crucial for smart business management; it allows you to optimize every aspect, from resource allocation to strategic planning. A key area where this shines is marketing, and crafting a winning strategy requires careful analysis. Check out this guide on developing a successful marketing plan for small business growth to understand how data informs effective campaigns.
Ultimately, leveraging data ensures your marketing efforts directly contribute to your bottom line, solidifying the importance of data-driven decision-making.
Data-Driven Marketing Strategies
Effective marketing hinges on understanding the customer. Data analysis provides invaluable insights into consumer behavior, preferences, and purchasing patterns. By leveraging data from website analytics, social media engagement, and CRM systems, marketers can create highly targeted campaigns, personalize messaging, and optimize ad spending. For instance, analyzing website traffic data can reveal which marketing channels are most effective, allowing companies to allocate resources accordingly.
A/B testing, driven by data on click-through rates and conversion rates, allows for continuous improvement of marketing materials and campaigns. Furthermore, predictive analytics can forecast future trends and customer behavior, enabling proactive marketing strategies. Imagine a clothing retailer using purchase history and browsing data to predict upcoming seasonal demands and adjust inventory levels accordingly, minimizing waste and maximizing profits.
Data Analysis in Supply Chain Management
Supply chain management is a complex network of processes, and data analysis is crucial for optimizing its efficiency. Real-time data tracking of inventory levels, transportation routes, and supplier performance enables companies to identify bottlenecks, predict potential disruptions, and proactively manage risks. For example, analyzing historical data on delivery times and weather patterns can help predict potential delays and implement contingency plans.
Predictive analytics can forecast demand fluctuations, enabling better inventory management and reducing storage costs. By analyzing data on supplier performance, companies can identify reliable partners and negotiate better terms. This data-driven approach leads to reduced costs, improved on-time delivery, and enhanced customer satisfaction.
Data’s Role in Enhancing Customer Relationship Management (CRM)
CRM is all about building and maintaining strong customer relationships. Data plays a pivotal role in personalizing the customer experience and improving customer satisfaction. Analyzing customer data, including purchase history, interaction logs, and feedback, allows businesses to understand customer needs and preferences. This enables personalized marketing campaigns, targeted offers, and proactive customer service. For example, a company can use data to identify high-value customers and offer them exclusive benefits or personalized support.
Analyzing customer feedback can help identify areas for improvement in products or services. By leveraging data, businesses can build stronger customer loyalty and increase lifetime customer value.
Integrating Data Insights into Human Resource Management
Data-driven decision-making significantly impacts human resource management (HRM). Analyzing employee data, such as performance reviews, training records, and attrition rates, can help HR professionals make informed decisions about recruitment, training, and compensation. For example, analyzing employee turnover data can help identify factors contributing to employee attrition, allowing HR to implement strategies to improve employee retention. Performance data can be used to identify high-performing employees and tailor development plans to enhance their skills.
Data analysis can also help ensure fair and equitable compensation practices by identifying potential biases in salary structures. This data-driven approach to HRM fosters a more engaged and productive workforce.
Overcoming Challenges in Data-Driven Decision Making
Embracing data-driven decision-making isn’t a simple switch; it’s a journey fraught with obstacles. Successfully navigating this path requires acknowledging and proactively addressing the challenges that can hinder progress. From ensuring data privacy to fostering a culture of data literacy, overcoming these hurdles is crucial for reaping the full benefits of data-driven insights.Data privacy and security concerns are paramount in today’s business environment.
The increasing reliance on data necessitates robust security measures to protect sensitive information from unauthorized access, breaches, and misuse. This includes adhering to regulations like GDPR and CCPA, implementing strong encryption protocols, and establishing clear data governance policies. Failure to prioritize data security can lead to significant financial losses, reputational damage, and legal repercussions.
Data Privacy and Security Concerns
Organizations must implement comprehensive data security measures to protect sensitive information. This involves establishing robust access control systems, encrypting data both in transit and at rest, and regularly conducting security audits to identify and address vulnerabilities. Investing in advanced security technologies, such as intrusion detection systems and firewalls, is crucial for mitigating the risk of data breaches. Furthermore, employees need thorough training on data security best practices, including password management, phishing awareness, and safe data handling procedures.
Compliance with relevant data privacy regulations, such as GDPR and CCPA, is also essential to avoid penalties and maintain customer trust. Consider a hypothetical scenario where a company experiences a data breach resulting in the exposure of customer credit card information. The financial and reputational consequences could be devastating, impacting not only the company’s bottom line but also its long-term viability.
Data Bias Mitigation
Data, inherently, can reflect existing biases present in the society or processes from which it is collected. These biases, if left unaddressed, can lead to flawed insights and unfair or discriminatory outcomes. For example, algorithms trained on historical hiring data might perpetuate gender or racial biases, leading to unequal opportunities. Mitigating these biases requires careful data selection, rigorous data validation, and the use of fairness-aware algorithms.
This involves critically evaluating data sources for potential biases, employing techniques like data augmentation to balance datasets, and using algorithms designed to minimize discriminatory outcomes. Regular audits of algorithms and models are crucial to identify and correct any emerging biases.
Data Literacy and Training
Data literacy is not just about understanding numbers; it’s about interpreting data effectively to make informed decisions. Equipping employees with the necessary data literacy skills is paramount to a successful data-driven strategy. Comprehensive training programs should cover data interpretation, visualization, and analysis techniques. This empowers employees to understand data insights, contribute effectively to data-driven discussions, and ultimately, drive better business outcomes.
Imagine a scenario where a marketing team lacks the skills to interpret website analytics. Their decisions regarding marketing campaigns would be based on intuition rather than data, potentially leading to wasted resources and ineffective campaigns.
Best Practices for Overcoming Resistance to Data-Driven Decision Making
Successfully implementing data-driven decision-making requires addressing potential resistance from employees who may be accustomed to traditional methods. Building a supportive culture and demonstrating the value of data are key.
- Clearly Communicate the Benefits: Showcase tangible examples of how data-driven decisions have improved business outcomes.
- Provide Comprehensive Training: Equip employees with the necessary data literacy skills and tools.
- Foster Collaboration: Encourage cross-functional collaboration to integrate data insights across departments.
- Celebrate Successes: Highlight instances where data-driven decisions have yielded positive results.
- Address Concerns Openly: Create a safe space for employees to voice their concerns and address any resistance proactively.
- Start Small and Scale Gradually: Begin with pilot projects to demonstrate the value of data-driven decision-making before implementing it company-wide.
Measuring the Impact of Data-Driven Decisions: The Importance Of Data-driven Decision Making In Business Management
So, you’ve embraced data-driven decision-making. Fantastic! But how do you know if it’s actually working? Simply implementing data analysis isn’t enough; you need to measure the impact to justify the investment and demonstrate its value to stakeholders. This section explores key performance indicators (KPIs) and methods for tracking the return on investment (ROI) of your data-driven initiatives.Measuring the success of data-driven decision-making requires a strategic approach.
It’s not just about crunching numbers; it’s about linking those numbers to tangible business outcomes and demonstrating a clear connection between data-informed choices and improved performance.
Key Performance Indicators (KPIs) for Data-Driven Strategies
Effective measurement hinges on selecting the right KPIs. These metrics should directly reflect the goals of your data-driven initiatives. For example, if your goal is to improve customer retention, KPIs like customer churn rate, customer lifetime value (CLTV), and Net Promoter Score (NPS) become crucial. If your focus is on operational efficiency, you might track metrics such as production cycle time, defect rate, or inventory turnover.
The choice of KPIs depends entirely on the specific business objectives and the data available.
Tracking and Measuring the Return on Investment (ROI) of Data-Driven Initiatives
Calculating the ROI of data-driven initiatives requires a clear understanding of both the costs and the benefits. Costs include the investment in data infrastructure, software, personnel, and training. Benefits, on the other hand, are often more complex to quantify and might include increased revenue, reduced costs, improved efficiency, enhanced customer satisfaction, or minimized risks. A simple ROI calculation can be represented as:
ROI = (Net Benefit – Total Cost) / Total Cost – 100%
For instance, if a company invests $10,000 in a new data analytics system that leads to a $25,000 increase in revenue and a $5,000 reduction in operational costs, the ROI would be: (($25,000 + $5,000)
- $10,000) / $10,000
- 100% = 200%. This illustrates a significant return on the investment. However, accurately attributing the entire benefit solely to the data analytics system can be challenging, and a more nuanced approach might be required.
Demonstrating the Positive Impact of Data-Driven Decisions on Business Outcomes
Demonstrating the positive impact isn’t just about numbers; it’s about telling a compelling story. This involves presenting data visualizations, case studies, and narratives that clearly connect data-driven decisions to improved business outcomes. For example, showing how a targeted marketing campaign, informed by customer segmentation data, resulted in a significant increase in conversion rates provides a strong, tangible example of success.
Similarly, demonstrating how predictive maintenance, based on sensor data analysis, reduced equipment downtime and maintenance costs offers compelling evidence of the value of data-driven decision-making.
A Framework for Reporting on the Success of Data-Driven Decision-Making Projects
A robust reporting framework is essential for tracking progress, identifying areas for improvement, and demonstrating the overall value of data-driven initiatives. This framework should include:* Clearly defined objectives: What were you trying to achieve?
Key performance indicators (KPIs)
How will you measure success?
Data sources
Where did the data come from?
Methodology
How did you analyze the data?
Results
What were the outcomes?
Recommendations
What are the next steps?Regular reporting, using dashboards and visualizations, allows for continuous monitoring and adjustments to strategies, ensuring that data-driven initiatives remain aligned with business goals and deliver maximum impact. This framework allows for consistent evaluation and refinement of the data-driven approach, fostering continuous improvement.
The Future of Data-Driven Decision Making in Business
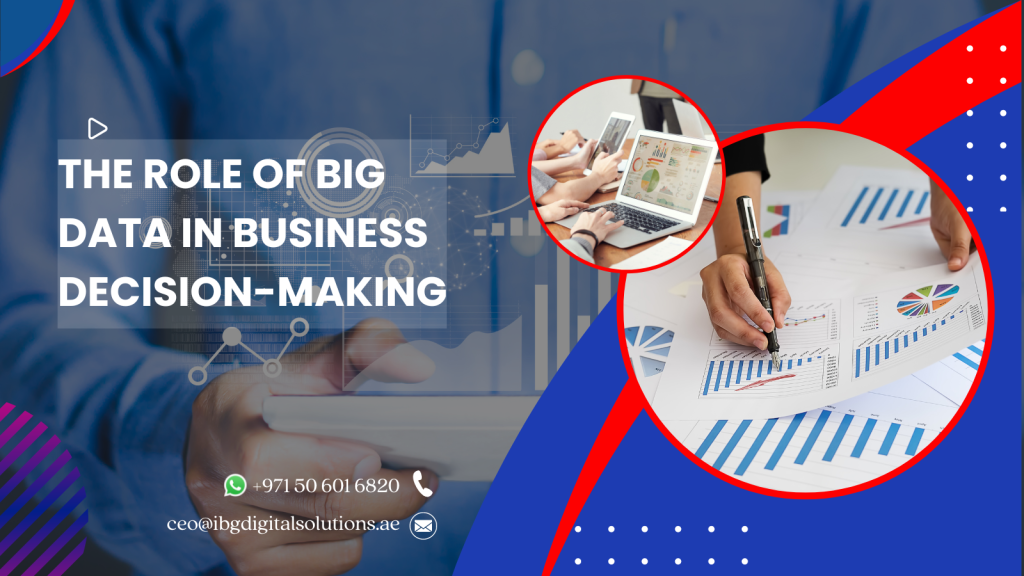
Data-driven decision-making is no longer a futuristic concept; it’s the present and the future of successful business management. As technology continues to evolve at an unprecedented pace, the ways businesses leverage data for strategic advantage will undergo a significant transformation. This section explores the emerging trends shaping the future of data-driven decision-making, the ethical considerations involved, and the transformative potential of artificial intelligence.
Emerging Technologies and Their Impact on Data Analysis and Decision-Making
The convergence of several technologies is revolutionizing data analysis and decision-making. Advanced analytics, including machine learning and deep learning algorithms, are enabling businesses to extract far more valuable insights from their data than ever before. For instance, predictive modeling, powered by machine learning, allows businesses to anticipate customer behavior, optimize supply chains, and mitigate risks more effectively. Real-time data streaming and processing technologies enable immediate responses to market changes and customer needs, leading to faster and more agile decision-making.
The Internet of Things (IoT) generates massive amounts of data from connected devices, providing granular insights into operational efficiency and customer usage patterns. This data, when analyzed effectively, can lead to significant improvements in product design, service delivery, and resource allocation. Consider, for example, a manufacturing company using IoT sensors to monitor equipment performance in real-time. Predictive maintenance based on this data can prevent costly downtime and optimize production schedules.
Future Trends in Data-Driven Business Management, The importance of data-driven decision making in business management
Several key trends are poised to shape the future of data-driven business management. One significant trend is the increasing importance of data democratization – making data accessible and understandable to a wider range of employees, not just data scientists. This empowers more individuals to contribute to data-driven decision-making, fostering a more data-literate and agile organization. Another key trend is the rise of augmented analytics, which uses AI to automate data preparation, analysis, and insight generation, making the process faster and more efficient.
This will free up data scientists to focus on more complex analytical tasks and strategic initiatives. Furthermore, we can anticipate a growing focus on ethical and responsible data use, driven by increasing regulatory scrutiny and heightened public awareness of data privacy concerns. Businesses will need to prioritize transparency, accountability, and fairness in their data practices. Finally, the integration of data-driven insights across all business functions will become increasingly critical for holistic and effective decision-making.
A company that successfully integrates data-driven insights across sales, marketing, operations, and finance will have a significant competitive advantage.
Ethical Considerations of Using Data in Business Decision Making
The use of data in business decision-making raises several crucial ethical considerations. Data bias, for example, can lead to unfair or discriminatory outcomes if not carefully addressed. Algorithms trained on biased data will perpetuate and amplify those biases in their predictions and recommendations. Businesses must actively work to identify and mitigate biases in their data and algorithms to ensure fairness and equity.
Data privacy is another major concern. Businesses must comply with relevant regulations, such as GDPR and CCPA, and implement robust data security measures to protect customer data. Transparency in data usage is also essential. Customers should be informed about how their data is being collected, used, and shared. Finally, the potential for data manipulation and misuse necessitates strong ethical guidelines and oversight to ensure the integrity and trustworthiness of data-driven decisions.
A company that fails to address these ethical considerations risks reputational damage, legal penalties, and loss of customer trust.
The Potential of Artificial Intelligence to Enhance Data-Driven Decision-Making Capabilities
Artificial intelligence (AI) has the potential to dramatically enhance data-driven decision-making capabilities. AI-powered systems can automate complex analytical tasks, identify patterns and insights that would be impossible for humans to detect, and provide real-time recommendations based on dynamic data streams. For example, AI can be used to personalize marketing campaigns, optimize pricing strategies, and improve customer service. AI can also help businesses to identify and manage risks more effectively by analyzing large datasets and identifying potential threats.
Furthermore, AI can facilitate more collaborative and inclusive decision-making by providing insights and recommendations to a wider range of stakeholders. However, it is crucial to remember that AI is a tool, and its effectiveness depends on the quality of the data it is trained on and the ethical considerations discussed earlier. A responsible and thoughtful approach to implementing AI in data-driven decision-making is essential for achieving positive outcomes.